Get smarter with your data.
Industry leading tools and services to solve business problems
Whether you’re a startup, SME or larger enterprise, we exist to assist with your data warehousing projects, helping to convert, manage and analyse your data more effectively.
Book a Demo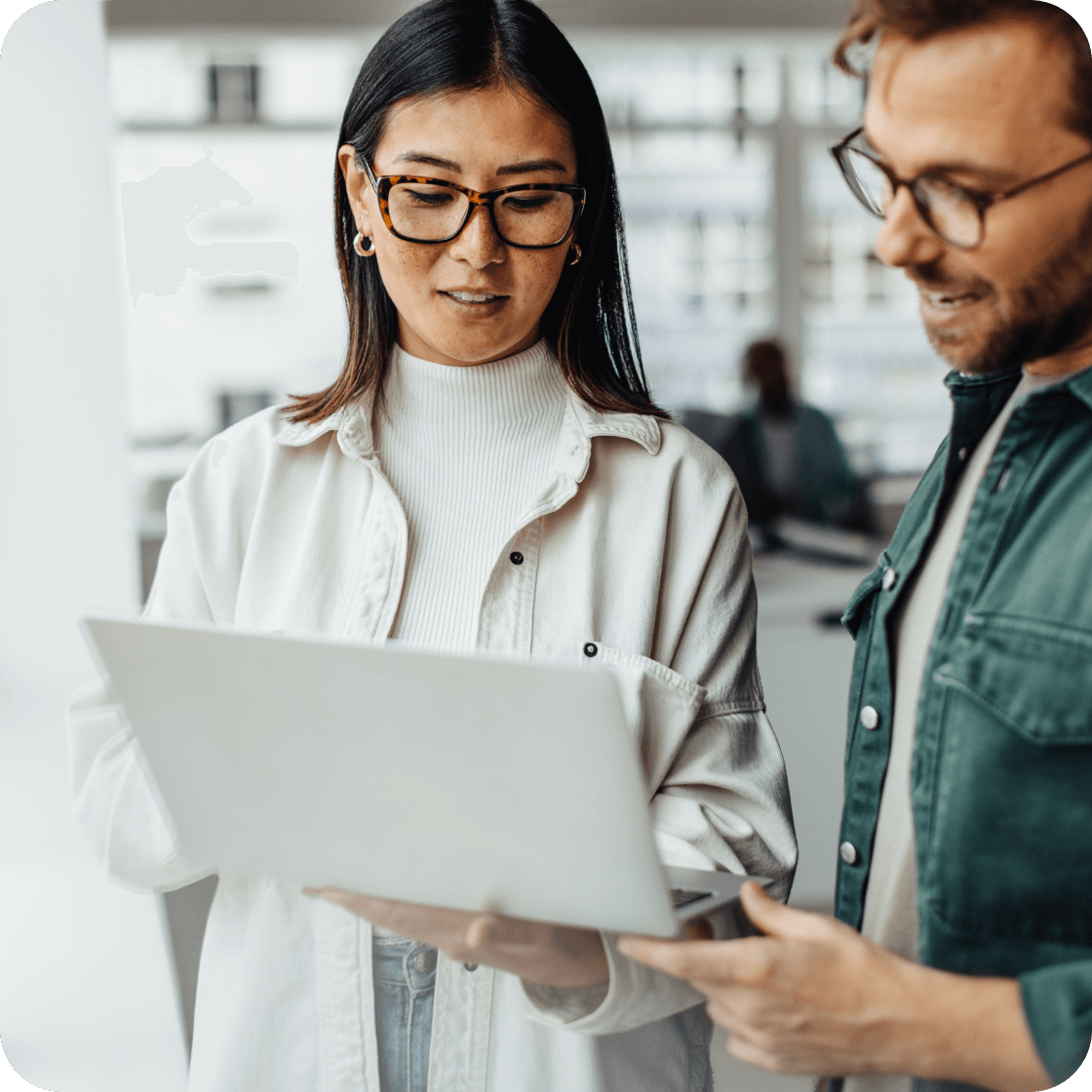
Trusted by:
Advice and tools that get your data up and running
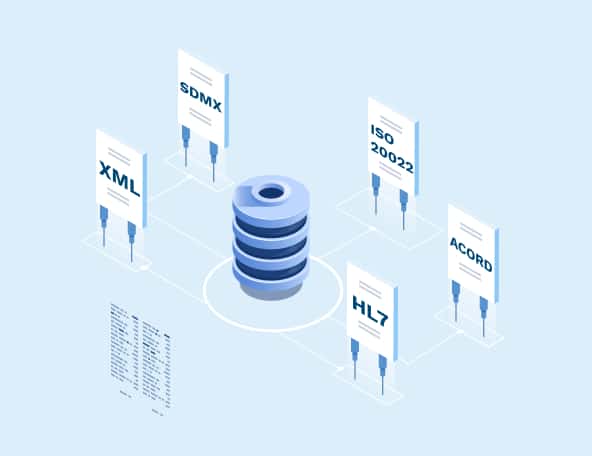
Flexter – Convert any XML to any format in seconds
Flexter is an enterprise-level data warehouse automation tool for industry data standards (XML/JSON). It requires no coding skills and automatically un-siloes industry-standard data and converts it into a readable format…
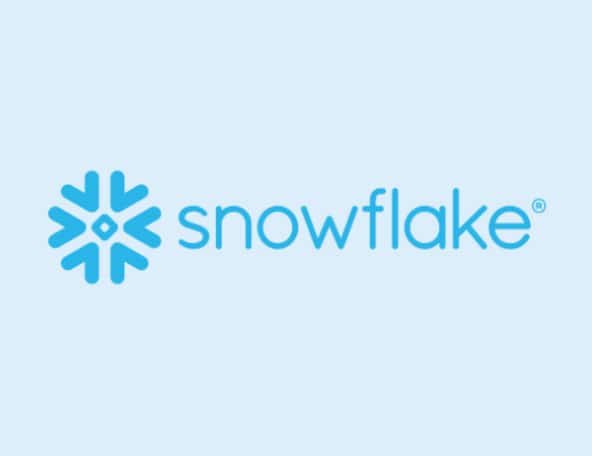
Data warehouse implementation services on Snowflake
With years of experience in implementing and managing Snowflake cloud data warehouse projects, we can help you migrate to the platform or fine-tune your existing setup.
As well as advising on the strategy, we can provide practical assistance to optimise your setup…
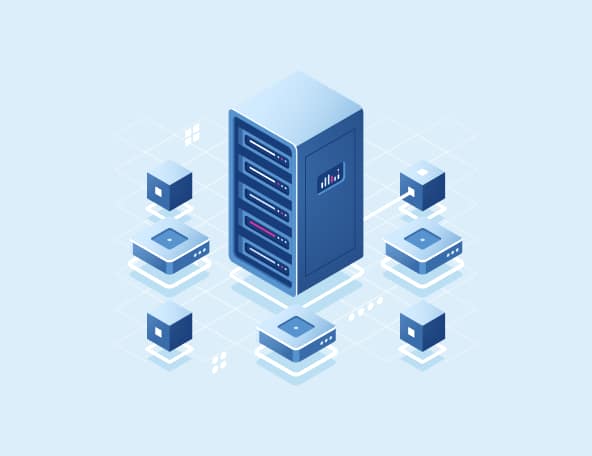
Data Warehouse & Data Lake Architecture Advisory
Whether you’re an established industry leader with a data lake architecture project or an organisation in need of modernizing your data management, we can advise you and guide your project towards a successful outcome…
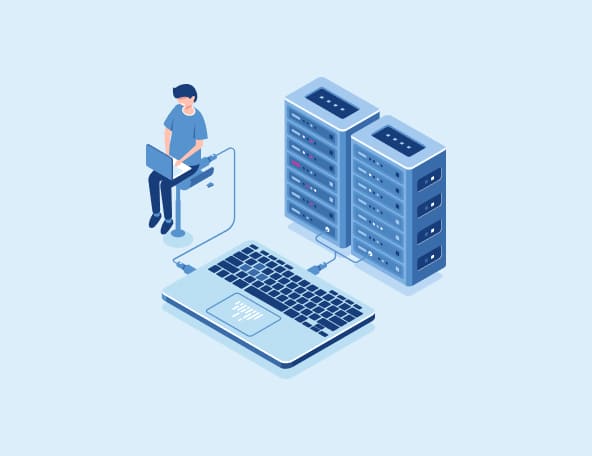
Health checks, mentoring, and more….
We can audit your existing data warehouse implementation to ensure that you’re following best practices and, if you’re a data warehouse leader, our mentors will work alongside you to help you overcome key challenges…
Who are we?
Anyone who has worked for a while in the rapidly changing data warehouse industry knows how hard it is to keep up.
When you can’t get the answers from existing data solutions, sometimes you have to create your own.
That’s why it helps to have a veteran of the industry on your team.
Uli Bethke is the founder and CEO of Sonra. He has implemented dozens of data warehouses across four continents, ranging from small data marts to large scale enterprises with multi-million-dollar budgets.
Uli is the author of several books on data integration and a regular speaker at international conferences. He is also a VP and the co-founder of DAMA Ireland, the Irish branch of DAMA International.
As well as assembling an expert team of data management advisors to work with organisations around the world, Uli is an innovator.
At Sonra, we have developed an industry-leading data conversion tool in Flexter.
You did in one day what we could not achieve in three years!
As the second biggest airline in Ireland, with over 10 million passengers annually, Aer Lingus must process enormous amounts of data.
The main issue was the nightly loading of booking data, which a project outsourced to a third party had failed to solve after 50 days.
We implemented Flexter to handle this. It required no custom development and was up and running within a day.
Key results:
- Eliminated project risk – Aer Lingus knew immediately that our solution worked.
- A dramatic cut in costs of XML ingestion – 120 man-days and the ongoing cost for code maintenance were eliminated.
- Meeting SLAs – Flexter performed orders of magnitude better than the previous aborted attempt, helping Aer Lingus meet SLAs.
What can you expect from us?
Whether you’re a data warehouse manager, a data architect, a data analytics manager, a director or a VP, the team at Sonra wants to make your life easier and more stress-free, while helping you improve performance in the organisation.
Whatever sector you operate in, we want you to get the kudos so that you can advance your career.
You can expect:
- Access to leading-edge technology that you may not even know exists
- Recommendations for saving time and reducing costs
- Seasoned advice based on a wealth of data warehousing experience
- Excellent communication every step of the way
- Gold-standard service
We often assist companies in finance, healthcare, travel, retail, and the automotive industry with notoriously slow, laborious and expensive data management issues.
However, we have the experience to solve your data problems regardless of the industry you are in.