How to convert XML to Spark Delta Tables and Parquet
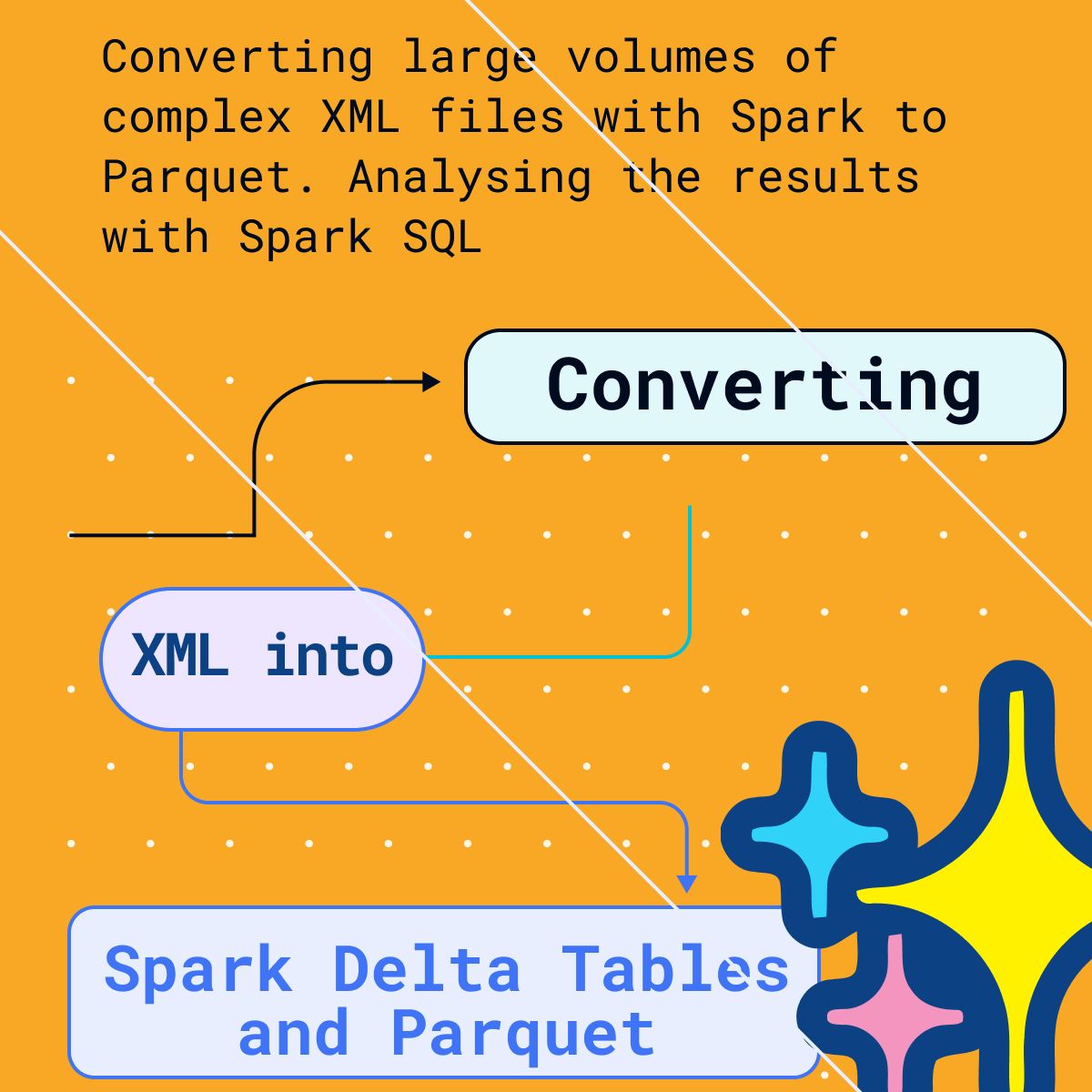
The main option for converting XML on Spark to Parquet and Delta Tables is the Spark XML-Library. It is an external library that can be integrated with Spark but does not ship with Spark natively.
In this blog post we will explore the limitations of the Spark XML-Library in particular and the manual coding approach of converting XML to Parquet or Delta Tables in general.
Spark-XML is not integrated directly with Databricks either. It is a separate install.
There are plans to ship the Spark XML library inside Spark 4.0.
We will present an alternative approach that is fully automated, outline its benefits and explain when to use the manual versus the automated approach.
The limitations of the Spark-XML library
The Spark XML-Library has various downsides
Manual coding: Using the Spark-XML library relies on a manual coding approach. It is time consuming and may delay your project. For complex scenarios based on industry data standards we have seen many projects fail entirely.
Denormalisation: The Spark XML library does not convert the XML hierarchy into a normalised representation of the data. For very simple XML files with a single branch this may be ok. However, if you have slightly more complex XML files then this will be an issue. As an analogy, think of dumping a complex ERP or CRM system with hundreds of tables into one flat table.
The library doesn’t accept multiple tables, it can’t handle complex trees, and it can’t work with an unknown xml tag structure.
XSD support: Spark-XML does not provide support for XSDs apart from very basic scenarios. It will not work with complex XSDs based on industry data standards.
Handling large XML documents: Spark-XML has problems handling very large XML files as they can not be split and processed across multiple nodes.
Schema evolution: Changes to XML files or unexpected XPaths are not handled gracefully, e.g. deleting or adding an attribute is not handled. Comparing different versions of XML is hard and requires manual intervention.
Handling XML versions: Working with different versions of a schema or XSD has to be handled manually, which may require significant refactoring. unified schema across multiple different versions of an XML schema is not handled.
The XML conversion life cycle
The process of converting XML data to Parquet or Delta Tables involves several key steps, many of which can be automated to streamline the process:
Use Flexter to turn XML and JSON into Valuable Insights
- 100% Automation
- 0% Coding
Analysis of XML Structure and XSD: Initially, a data analyst examines the structure of various XML documents. For more complex projects, an XML Schema Definition (XSD) is often available to guide this analysis. If these documents adhere to an industry data standard, relevant documentation, which can span several hundred pages, will also be reviewed.
Data Modeling: The next step involves creating a relational target model.
Mapping XML to Data Model: This phase requires mapping individual XML elements to the corresponding relational schema to Spark Parquet files or Delta Tables, ensuring that XML data can be accurately transformed and stored.
XML Conversion: Using tools and methods provided by the Spark XML-Library, the actual conversion of XML data to the target schema on SQL Server is performed. This step transforms the XML data into a format suitable for storage and analysis in a relational database.
Error Handling and Logging: It’s essential to implement mechanisms for identifying and logging invalid XML documents. Additionally, setting up alerting for such errors ensures that any issues can be promptly addressed.
Documentation: Finally, documenting the entire process, including the code used, mappings between XML elements and database schema, the target data model, and any other relevant information, is critical. This documentation supports future maintenance and scalability of the conversion process.
By following these steps, organisations can effectively manage the transition from complex XML documents to a structured relational database system, making the data more accessible and usable for analysis and reporting purposes.
Automated versus manual XML conversion on Spark
Let’s have a look at how these steps compare between a manual XML conversion approach using coding and an automated approach using an XML conversion tool. For this comparison we use Flexter to illustrate the hands on steps.
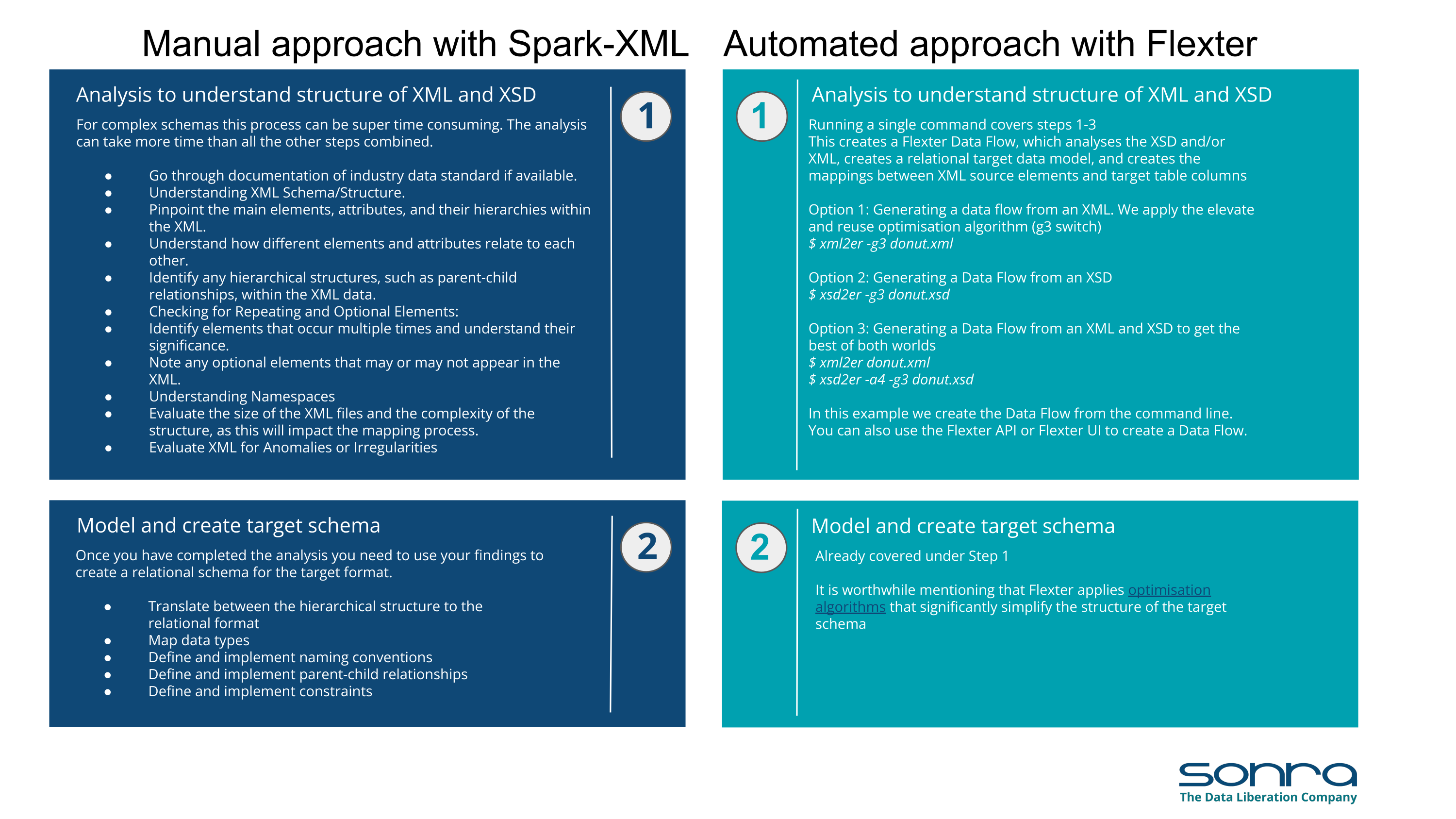
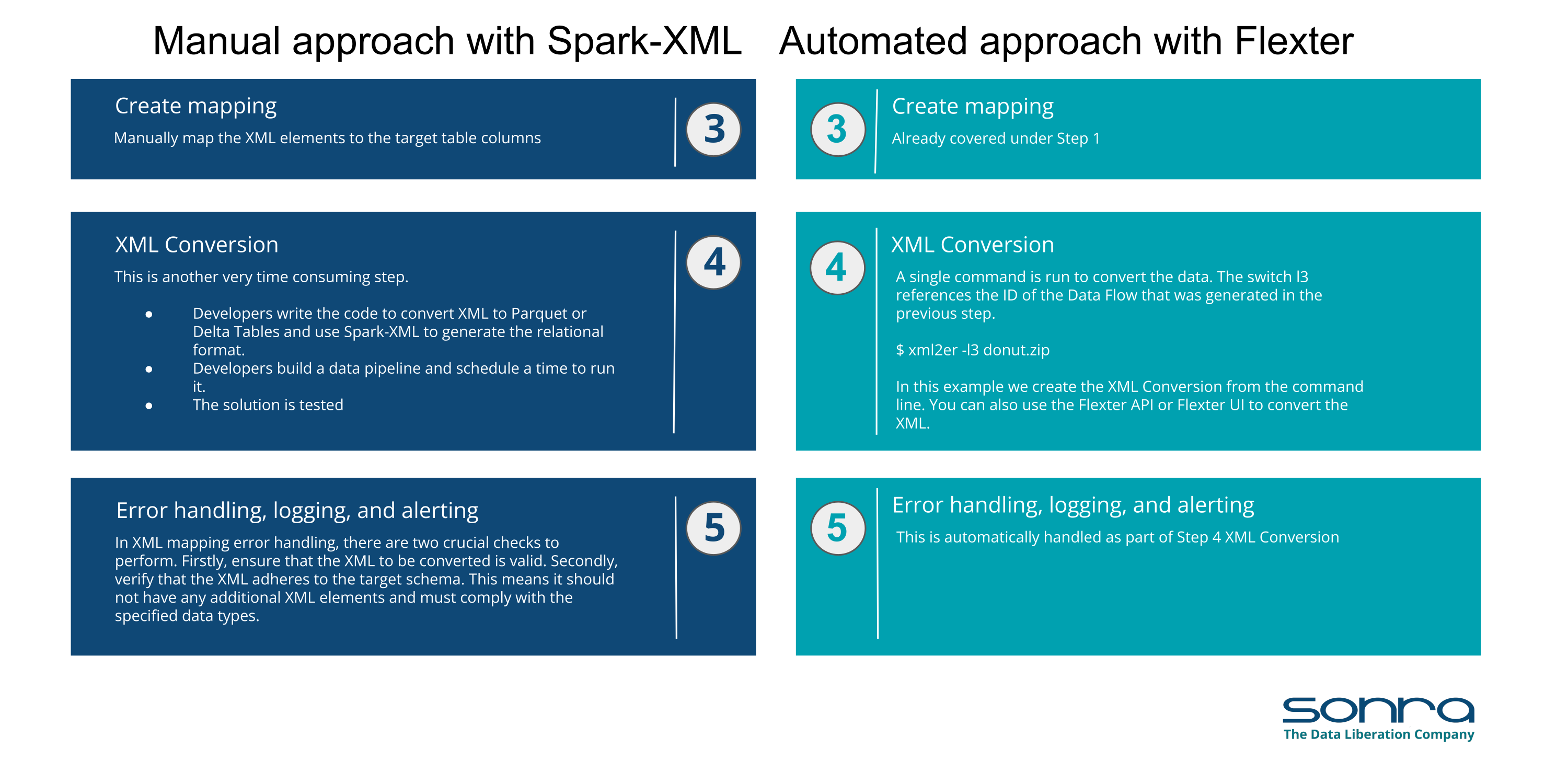
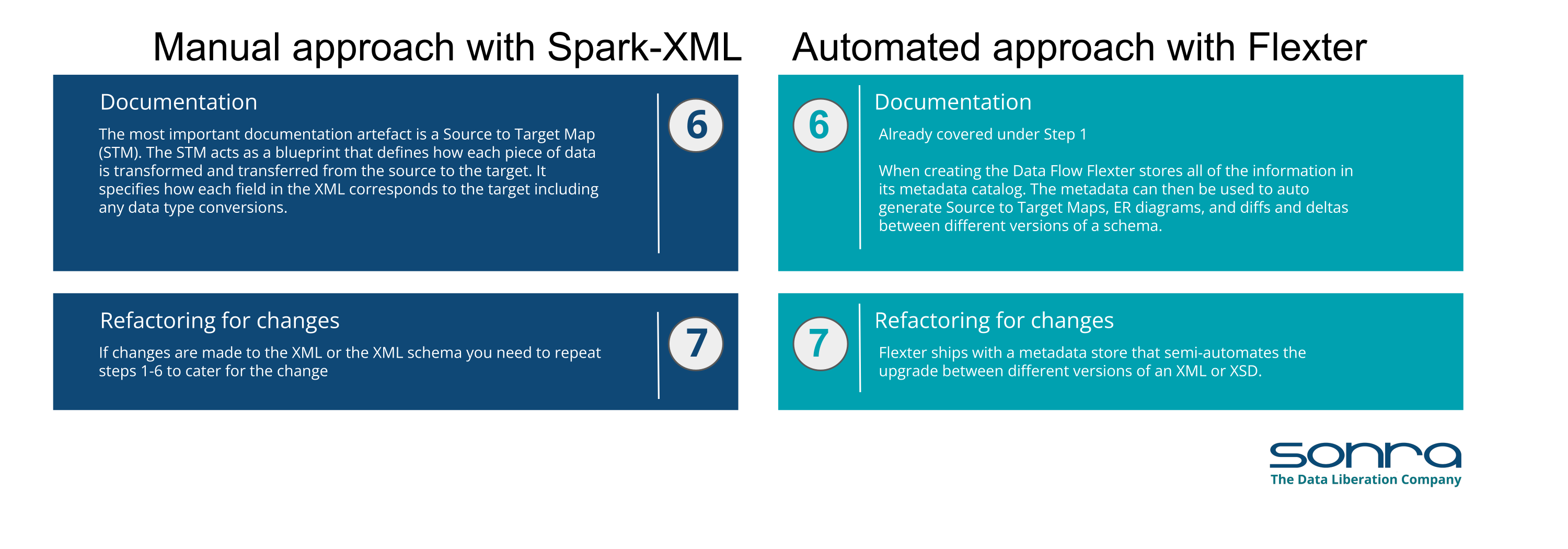
You also have the option to download a PDF that provides this side-by-side comparison of manual versus automated XML conversion.
The benefits of automated XML conversion on Spark
There are some clear benefits of using an automated conversion approach on Spark. The same applies to XML conversion on Databricks by the way.
Complexity? No problem. An automated setup zips through all kinds of XML and XSD complexities in no time.
Speedy Start: With automated XML parsing, all those steps like analysing, creating schemas, and mapping are done for you. This means data gets to decision-makers super fast.
Less Risky: Automating the XML parsing slashes the chances of projects going over budget or belly-up, especially those tricky ones based on industry standards.
Surefire Consistency and Spot-On Accuracy: Automation keeps the XML parsing spotless and consistent, minimising the risk of human mistakes.
Top-Notch Performance: Need to ramp up for big XML data volumes or tight SLAs? An XML automation tool has your back, scaling up and out as needed.
Easy-Peasy: Automated tools come with user-friendly interfaces, making the whole process a breeze. Plus, you won’t have to scramble for folks with hard-to-find XML skills like XPath, XQuery, XSLT, dodging potential project failures.
Ralph Kimball, the pioneer behind dimensional modelling, made his insightful observations for very good reasons.
“Because of such inherent complexity, never plan on writing your own XML processing interface to parse XML documents.
The structure of an XML document is quite involved, and the construction of an XML parser is a project in itself—not to be attempted by the data warehouse team.”
Kimball, Ralph: The Data Warehouse ETL Toolkit. Practical Techniques for Extracting, Cleaning, Conforming, and Delivering Data
How Flexter can help
Flexter is an enterprise XML conversion software.Flexter automatically converts complex XML to big data file formats (Delta Table, Parquet, Avro, ORC), Text (CSV, TSV etc.), or a database (Oracle, SQL Server, PostgreSQL etc.). You don’t have to write a single line of code. Everything happens automagically and you will be up and running in a day or two. It scales up on multiple CPUs and out on multiple servers..
If you want to find out more about Flexter visit the product pages or try automated XML conversion for free.
Let’s get started
In this example we will use Flexter to convert an XML file to parquet. We then query and analyse the output with Spark.
How does Flexter generate the target schema?
We generate the target schema based on the information from the XML, the XSD, or a combination of the two. If you can’t provide an XSD we generate the target schema from a statistically significant sample of the XML files. In summary you have three options to generate the target: (1) XML only (2) XSD only (3) Combination of XML and XSD.
When we generate the target schema we also provide various optional optimisations, e.g. we can influence the level of denormalisation of the target schema and we may optionally eliminate redundant reference data and merge it into one and the same entity.
Flexter can generate a target schema from an XML file or a combination of XML and XML schema (XSD) files. In our example we process airline data based on the OTA standard. Both the XML files and the XSD are available and we use the information from both files to generate the target schema.
1 2 3 4 5 6 7 8 9 10 11 12 13 |
# We first test that the XML file is well formatted by simulating the execution the skip switch (-s) $ xml2er -s data.xml # Next we extract the statistics from data.xml. Statistics are used to generate the target schema. We use the xml2er command line tool without the skip (-s) switch. $ xml2er data.xml … # The result of this operation is an ID (origin: 5). We will use this ID in subsequent steps origin: 5 job: 6 # Some useful execution statistics startup: 3717 ms parse: 752 ms stats: 6403 m Map: 3 ms |
Now that we have gathered statistics from our XML sample we can generate the logical target schema with the xsd2er command line tool using the -k switch (-k is shortcut for –use-stats)
1 |
-k, --use-stats <ID[,ID2..]> Use the stats to generate the new schema |
Let’s go through the steps
1 2 3 4 5 6 7 8 9 10 11 12 13 14 15 16 17 18 19 |
# Template $ xsd2er -s -k<XML Schema ID> -g<Optimization Level> INPUTPATH # We first simulate generating the target schema with -s skip switch $ xsd2er -s -k5 -g3 schema.xsd # everything worked. Now running the command for real without skip $ xsd2er -k5 -g3 schema.xsd … # schema origin: 6 logical: 4 job: 8 # statistics startup: 444 ms stats: 53 ms parse: 670 ms build: 229 ms write: 47 ms map: 334 ms xpaths: 207 |
Happy days. Now we use the Logical Schema ID (origin: 6) to convert the XML data to Parquet
1 2 |
# First simulating the conversion process $ xml2er -s -l4 data.xml |
When the command is ready, removing –skip or -s, allows us to process the data. We direct the parquet output to the output directory for the data.xml file. Let’s first create a folder “output_dir” as the location to extract the generated output. The location is given by -o parameter when extracting data using xml2er command.
1 2 3 4 5 6 7 8 9 10 11 12 13 14 15 |
$ mkdir output_dir $ xml2er -l4 -o root/output_dir/ -f parquet -z none -S o data.xml … 17:16:24.110 INFO Finished successfully in 17701 milliseconds # schema origin: 7 logical: 4 job: 9 # statistics startup: 2899 ms load: 7549 ms parse: 179 ms write: 5470 ms stats: 1083 ms xpaths: 207 |
We can find the extracted parquet files in the output folder. It is a directory structure, which you can find in the current directory. We can ‘ls’ to see the contents of the .parquet folder as shown below.
1 2 3 4 5 6 7 8 9 10 11 12 13 |
# Looking at the parquet files generated $ ls -l total 92 drwxr-xr-x 2 root root 4096 Jan 7 18:39 AirTraveler.parquet drwxr-xr-x 2 root root 4096 Jan 7 18:38 Tax.parquet drwxr-xr-x 2 root root 4096 Jan 7 18:39 Telephone.parquet drwxr-xr-x 2 root root 4096 Jan 7 18:39 Ticketing.parquet drwxr-xr-x 2 root root 4096 Jan 7 18:39 TravelerRefNumber.parquet … # Looking inside a parquet folder $ cd Ticketing.parquet $ ls part-00000-6e378cb3-bf61-41cc-ab1a-92cb12e0368f.parquet _SUCCESS |
In order to look inside the parquet files, let’s initiate the spark-shell and create a dataframe to load the parquet tables parsed using Flexter
1 2 3 4 5 6 7 8 9 10 11 12 13 14 |
$ spark-shell Spark context Web UI available at http://172.17.0.2:4041 Spark context available as 'sc' (master = local[*], app id = local-1515355322712). Spark session available as 'spark'. Welcome to ____ __ / __/__ ___ _____/ /__ _\ \/ _ \/ _ `/ __/ '_/ /___/ .__/\_,_/_/ /_/\_\ version 2.1.1 /_/ Using Scala version 2.11.8 (OpenJDK 64-Bit Server VM, Java 1.8.0_151) Type in expressions to have them evaluated. Type :help for more information. scala> |
Once we have initiated the spark-shell, we can proceed with reading the parquet files generated and import them as dataframes in spark.
1 2 3 4 5 6 7 |
# Creating the dataframes using the parquet files scala> val df1 = spark.read.parquet("Tax.parquet") scala> val df2 = spark.read.parquet("Ticketing.parquet") scala> val df3 = spark.read.parquet("TravelerRefNumber.parquet") scala> val df4 = spark.read.parquet("PTC_FareBreakdown.parquet") scala> val df5 = spark.read.parquet("PaymentDetail.parquet") scala> val df6 = spark.read.parquet("AirTraveler.parquet") |
We can take a look at the schema of the data frames generated and do some preliminary analysis before proceeding further on the data parsed. For example, let’s look at the “Ticketing data” and the “Air Traveler data” created above.
1 2 3 4 5 6 7 8 9 10 11 12 13 14 15 16 17 |
# printing the Schema of the dataframes created above scala> df2.printSchema() root |-- PassengerTypeCode: string (nullable = true) |-- TicketDocumentNbr: string (nullable = true) |-- TicketingStatus: string (nullable = true) |-- TravelerRefNumber: decimal(2,0) (nullable = true) ... scala> df6.printSchema() root |-- Address_CountryName: string (nullable = true) |-- Email: string (nullable = true) |-- GroupInd: string (nullable = true) |-- PersonName_GivenName: string (nullable = true) |-- PersonName_Surname: string (nullable = true) |-- TravelerRefNumber: decimal(2,0) (nullable = true) ... |
We can see that headers and data types of the various columns. We can also perform some basic analysis on the dataset in Scala and look at the various variables present
1 2 3 4 5 6 7 8 9 10 11 12 13 14 15 16 17 18 19 20 21 22 23 24 25 26 |
# showing all the values of GroupInd column in df6 scala> df6.select("GroupInd").show() +--------+ |GroupInd| +--------+ | N| | N| | N| | N| | Y| | N| | N| | Y| | N| +--------+ # showing all the distinct values of TravelerRefNumber column in df2 scala> df2.select(df2("TravelerRefNumber")).distinct.show() +-----------------+ |TravelerRefNumber| +-----------------+ | 1| | 2| | 2| | 3| ... +-----------------+ |
Various basic data processing can be performed on the dataframe generated on the steps above as given below. The sql function on a SparkSession enables applications to run SQL queries programmatically and returns the result as a DataFrame.
1 2 3 4 |
# filtering the data frame based on the values of a certain column scala> df.filter($"<column-name>" > value).show() # group by the values of a column and creating a count scala> df.groupBy("<column-name>").count().show() |
Let’s take the df2 data frame which contains the Ticketing.parquet output and query the rows which contains the non-null values of the TravelerRefNumber.
Temporary views in Spark SQL are session-scoped and will disappear if the session that creates it terminates. If you want to have a temporary view that is shared among all sessions and keep alive until the Spark application terminates, you can create a global temporary view. Global temporary view is tied to a system preserved database global_temp, and we must use the qualified name to refer it, e.g. SELECT * FROM global_temp.view1.
1 2 3 4 5 6 7 8 9 10 11 12 13 14 |
# Creating a Global temporary view df1.createOrReplaceTempView("TaxTable") # Selecting rows containing positive values of the column Amount val qTicket = spark.sql("SELECT * FROM TaxTable where Amount > 0") # Displaying the output above qTicket.show() +--------------------+------+------------+-------+ |PTC_FareBreakdown|Amount|CurrencyCode|TaxCode| +--------------------+------+------------+-------+ |33000000000000000...| 36.4| CHF| CH| |33000000000000000...| 43.5| CHF| YQ| |33000000000000000...| 21.06| CHF| UP| ... +--------------------+------+------------+-------+ |
We can also perform other SQL queries on the dataframes. Let’s take an example to perform a join on the two datasets loaded from the parquet files
1 2 3 4 5 6 |
# loading Air traveler dataset Val AirTraveler=sqlContext.read.format("parquet").option("header","true").load("AirTraveler.parquet") # loading Ticketing dataset Val Ticketing=sqlContext.read.format("parquet").option("header","true").load("Ticketing.parquet") # Inner join on both the datasets on the common column TravelerRefNumber val AirTicket = AirTraveler.as('a).join(Ticketing.as('b), $"a.TravelerRefNumber" === $"b.TravelerRefNumber") |
When should you use an automated approach for converting XML?
We’ve shown how cool Flexter is at turning XML into Spark Parquet automatically. But let’s be real, Flexter isn’t the perfect fit for everything. When thinking about using an automated tool like Flexter, you’ve got to balance the cool perks against the extra dollars it costs.
If your needs are simple or you’re just dabbling with XML every now and then, you probably don’t need a fancy XML automation tool. But, here are some signs that XML conversion software like Flexter might just be what you need:
- Got super complicated XML that’s using an XSD or follows strict industry rules like HL7 or FpML?
- Need to deal with lots of different XML types?
- Working with XML files so big they’re practically novels?
- Need to chew through tons of XML data fast because of tight deadlines?
- Is your team more “XML? What’s that?” than “XML pros”?
- Are you working under tight deadlines for converting XML data?
If any of these apply to you, why not take Flexter for a spin with its free online version? See for yourself how it can make your life easier. Or, if you want to get down to the nitty-gritty, talk to one of our XML conversion experts about your use case.
Further Reading
Deep dive on XML converters, XML conversion tools and XML conversion projects
Converting XML and JSON to a Data Lake
How to Insert XML Data into SQL Table?
The Ultimate Guide to XML Mapping in 2024
Optimisation algorithms for converting XML and JSON to a relational format